How Knowledge Graphs Accelerate Pharma Drug Discovery
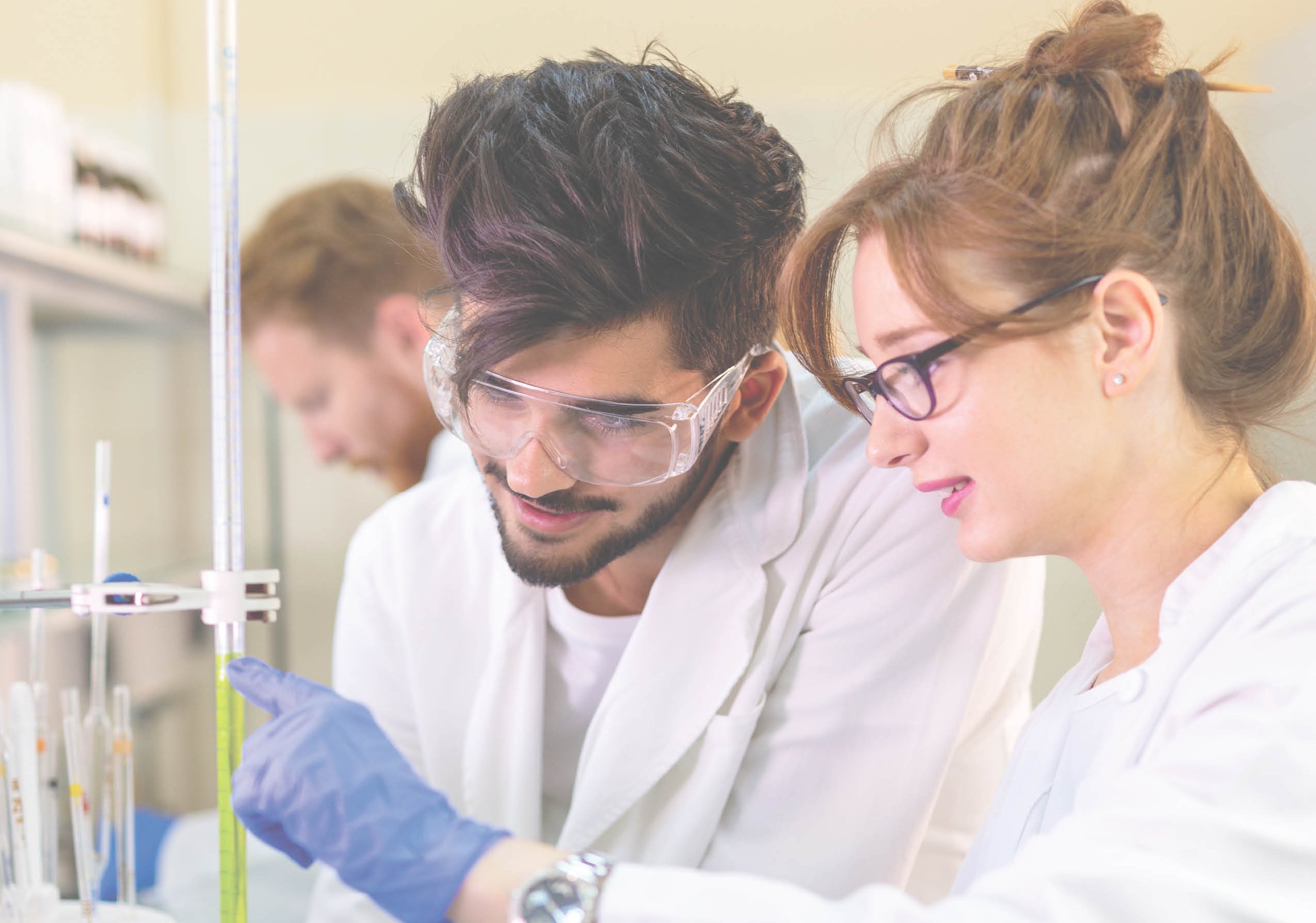
The pharmaceutical sector is a global trillion-dollar industry driven by constant change and innovation. It is also a heavily regulated industry and repeatedly comes under scrutiny when landmark medication is released. The heated global discussion about the Covid-19 vaccines has highlighted this.
Drug discovery is the process of new drug identification. This process is driven by leveraging the ever-increasing data from existing chemical libraries and data banks. It is estimated that a pharmaceutical company can take about 12 years to research and develop a new drug molecule with costs anywhere between 314 million to 2.8 billion dollars. Almost two-thirds of experimental targets fail either due to a lack of efficacy, compliance issues, competitor success, or simply because the chosen drug target itself is no longer viable.
The key to succeeding at drug discovery is to find a faster and more effective approach to data identification, usage, and repurposing. Data is abundant in the medical and pharma sector. Alas, data is siloed – not only between organizations but even within organizations. The nature of data has also changed with increasing amounts of unstructured data being ingested by companies. This makes it increasingly important for pharma companies to re-examine their data and research processes to optimize drug development.
Established Data Processes Are Sluggish & Expensive
Generally, drug engineers must manually collect, assess, relate and synthesis massive amounts of data from various data source to gain the relevant insights. Traditionally, an engineer must comb through internal databases, public databases, knowledge platforms, documents, articles, and spreadsheets to get the whole picture and to come up with a feasible drug target.
Given the complex relationships of data across multiple stages of trials and research, this process is frustratingly slow and cumbersome. High costs and risk of failure and errors are common.
Drug Discovery Is About Connecting the Dots
Knowledge graphs can be used to integrate medical data from different sources and apply automated reasoning processes. For example, connecting drugs, proteins, diseases, pathways, and other related information through semantic data relationships and visualizing that data mesh as a knowledge graph helps derive hidden and new insights. It helps companies to not only speed up the research process but also to reduce the errors and failure of hypotheses, thereby increasing the chance of success while reducing costs.
Knowledge Graphs have considerable value across a range of tasks, including drug repurposing, drug toxicity prediction and target gene-disease prioritization. In a knowledge graph-based representation of drug discovery domains, genes, diseases, and drugs are represented as entities or vertices. The connections or edges between them represent relations which can take any form (e.g. source, meaning, trail, compatibility, properties and characteristics or any other contextual relation imaginable). For example, an edge between a disease and drug entity might represent a successful clinical trial. Or an edge between two drug entities could indicate a potentially harmful interaction or compatibility. Engineers can explore those relations in a visual data map (i.e. “follow the track”) or via queries. That way, it is easy to gain new insights, browse trial histories and understand context.

The Future of Drug Discovery
eccenca Corporate Memory enables Pharma companies to solve their most pressing data and knowledge management challenges:
- Integrate high volumes of heterogenous data that is highly related but siloed.
- Infuse knowledge into data. This shifts the focus on applying knowledge to retrieve meaningful answers to solve real time queries effectively.
- Maximize the access and reuse of data. Data follows the FAIR principles-- findability, accessibility, interoperability, and reusability—to optimize the impact of data.
- Ensure a shared understanding of data in interdisciplinary, international, and multi-corporate teams
- Improve decision making by uncovering hidden/indirect relationships as well as constraints, context, and lineage.
- Simplify and automate data processes by leveraging AI for data mapping & knowledge infusion processes.
- Leverage contextual search and reasoning to answer complex questions.