How Knowledge Graphs Advance Diagnosis, Treatment and Sales in the Pharma Industry
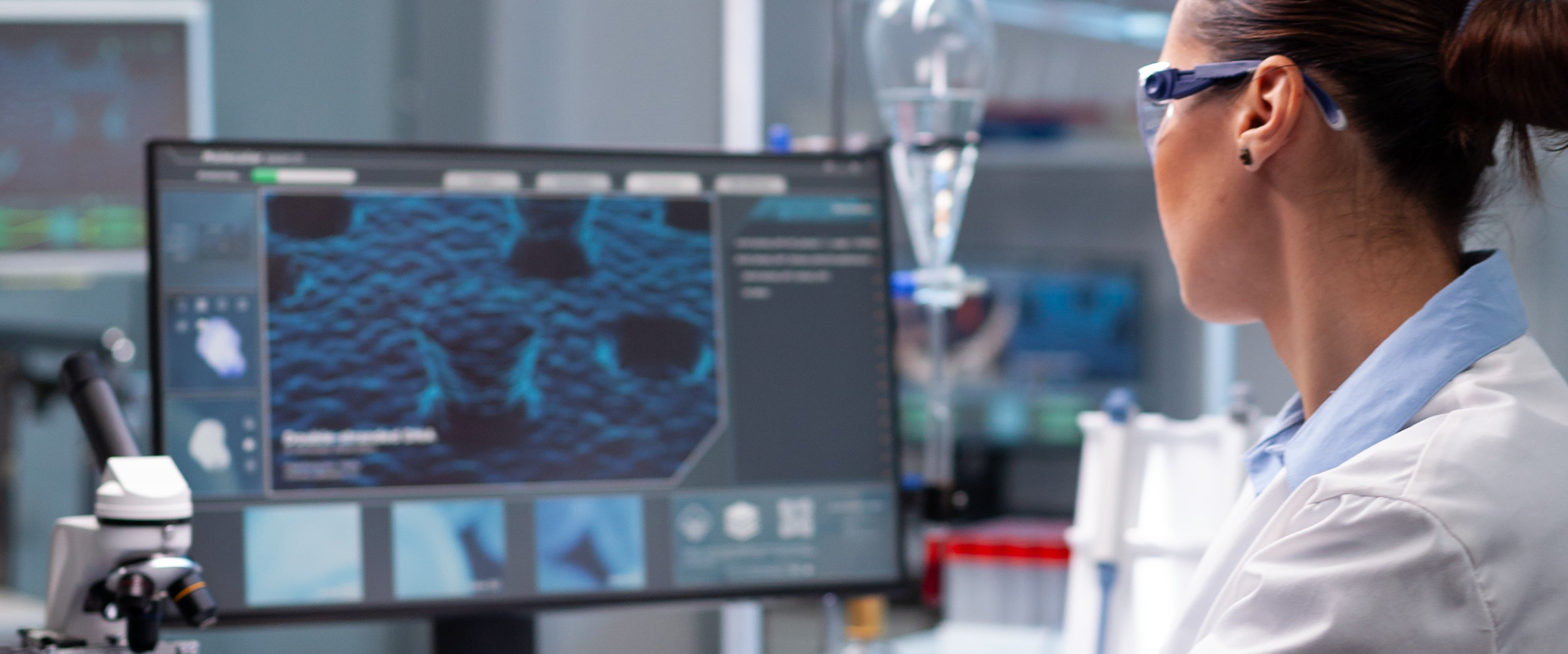
Studies have demonstrated that knowledge graphs – where artificial intelligence (AI) and machine learning (ML) are used – have brought about a sea of change in the pharmaceutical industry’s landscape by helping them sell better.
They are able to achieve them by making clinical and research trials more efficient, augmenting innovations, aiding in devising new tools for physicians and end-users, besides helping the healthcare industry to make more informed decisions. The data in circulation in the pharma industry is humongous. It is gathered by pharma companies and hospitals when they undertake researches, R&D tasks, clinical trials and results of outcomes of wellness through several years. Until the end of the 20th Century, data was, however, analyzed manually by healthcare specialists. But it bugged the pharma industry majorly by making data sharing and regulation difficult.
With drug makers partnering with IT companies, researchers and others, they end up sharing the healthcare data of large chunks of the population. Although there is a lack of authorized regulatory framework the world over to enable the pharma sector to embrace AI, some companies have managed to put in place governance measures for such partnerships, allowing this vertical, as a whole, to benefit.
Knowledge Networks Instead of Data Silos
Knowledge graphs (KGs), unlike relational databases, represent information in graphs. Here, entities (“nodes”) are pharmaceutical companies and hospitals that are linked in different lines (“edges”) to one another. Data, both structured and unstructured, mined from different and disparate sources will be gathered for processing and later to be assessed. Because of this type of representation, it is possible to process queries at astronomical speeds to get answers instantaneously.
Using KGs, the pharma industry has been able to accelerate the rate at which conditions are being diagnosed. The way diagnoses are being carried out has also improved. It is frustrating when the conditions of patients remain undiagnosed for prolonged periods. As the healthcare specialists remain unsuccessful at zeroing in on the right therapies, they wrest with various medical treatments to identify the right cures for problems. The major hurdle here is their helplessness to dig through past records of instances of treating patients with certain symptoms.
But KGs help pharma companies in tracking the medical histories of patients depending upon specific yardsticks, like symptoms, treatments, lab results, etc. This information will help in diagnosing conditions quickly and in an improved manner, tracking improvements and suggesting treatments to patients depending on their individual histories.
KGs also help healthcare professionals detect diseases before they strike patients based on their medical histories. In such cases, the right treatment can be recommended to them much earlier to prevent the outbreak of those ailments. Pharma companies, meanwhile, can use the same data to launch direct campaigns to advertise their medications and therapies. Alternately, they can build awareness where such conditions may be existing in certain untested people who may be having them. In addition to boosting the sales of the pharmaceutical companies, they also help them in identifying individuals who may be at risk.
Information about the progress of a patient helps in identifying whether certain medical treatments or effective or not. It can be then put to use by medical professionals to foretell the results of treatments in patients.
All Pharma Use Cases Depend On Exact & Contextual Data
Knowledge graphs aid healthcare professionals in devising roadmaps for treatments of patients afflicted with severe diseases too as they monitor their responses even when there are slightest changes in drug dosages, improving their experiences in achieving better health outcomes.
They help pharmaceutical companies in recording trends of physicians. It may well include the frequency of a certain treatment’s efficacy in treating patients, assessing medical procedures and detecting the basic needs of patients based on their respective environments. These companies may also use this data to conduct market research extensively on a commercial basis.
Data helps experts in gathering critical patient evidence in real-time so that medical professionals can take action immediately to avert occurrences of serious consequences. Patients have been monitored based on the risks they would have faced with the aid of equipment based on AI (including sensors, ECGs and other useful devices) to capture data and detect possible risks.
With massive data being accessible to both healthcare professionals and pharmaceutical players across fields of medicine, machine learning can be used to process it to identify the right physicians for patients suffering from different ailments in quick time. When this is achieved, the physicians can share those experiences in writing or through their speeches with their counterparts across the globe, allowing pharma companies to identify the right audiences for specific conditions.
As telemedicine is evolving at a disproportionate pace in the healthcare field, pharmaceutical companies are collaborating more with tech companies to develop online processes to monitor patients’ conditions, gather various treatment processes, improve regulations and also alert patients on time about their medical appointments. KGs also help medical professionals to virtually monitor if their patients are following their advice diligently or not.
In addition to gathering and storing data, they enable sharing of knowledge among healthcare professionals, improving the ability of the pharmaceutical industry to acquire more health-related information that will help them cut costs where required and to improve their R&D efforts on discovering new drugs, improving both their top and bottom lines eventually.
Find out how your Pharma company can leverage its data in our white paper "Intelligent Data Usage and Knowledge Capture in Drug Discovery".